Embodied Learning and Making in Design: In Dialogue with Machine Intelligence
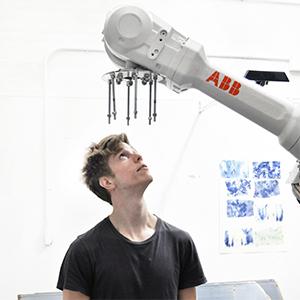
- Andrew Moorman, B.Arch. 2017
Hometown
Channahon, IllinoisClass
ARCH 5902 Design X: ThesisInstructor
Jenny Sabin
Don Greenberg
There is a current upsurge in mainstream generative design tools fortified by simulation and driving the concealed linear synthesis of optimized design alternatives faster. In pursuit of optimization, these tools saturate local machines or cloud servers with analysis and design iteration data, only to discard it once the procedure has concluded. Largely absent, however, are tools for an active, adaptive relationship with design exploration and the reuse of corresponding design data and metadata. In this thesis, it is proposed that these characteristics are mutually beneficial. The work presents a series of revisions to the optimization framework for routine design synthesis that examine a potential symbiosis between the production of large datasets (big data) and non-routine practices of making in design. Its engagement with iterative design exercises is twofold: as a supply of computer-generated design information to foster user intuition and explore the design space on non-objective terms, and as a supply of human-generated design information to learn artifacts of user preference in the interest of design software personalization. These concepts are applied to the generation of functionally graded patterning in chair design, combining methods of physical production with programmable sheet material behavior through a custom interactive synthesis framework.